Information about the Koyejo Group.
The Koyejo Group’s applied research is primarily in cognitive neuroimaging and biomedical imaging. Some recent highlights include:
- Generative models for biological images
- Graph estimation and analysis, particularly for time-varying brain graphs.
Our Principal Investigator is Sanmi Koyejo.
Check out the tabs below or our website here to learn more.
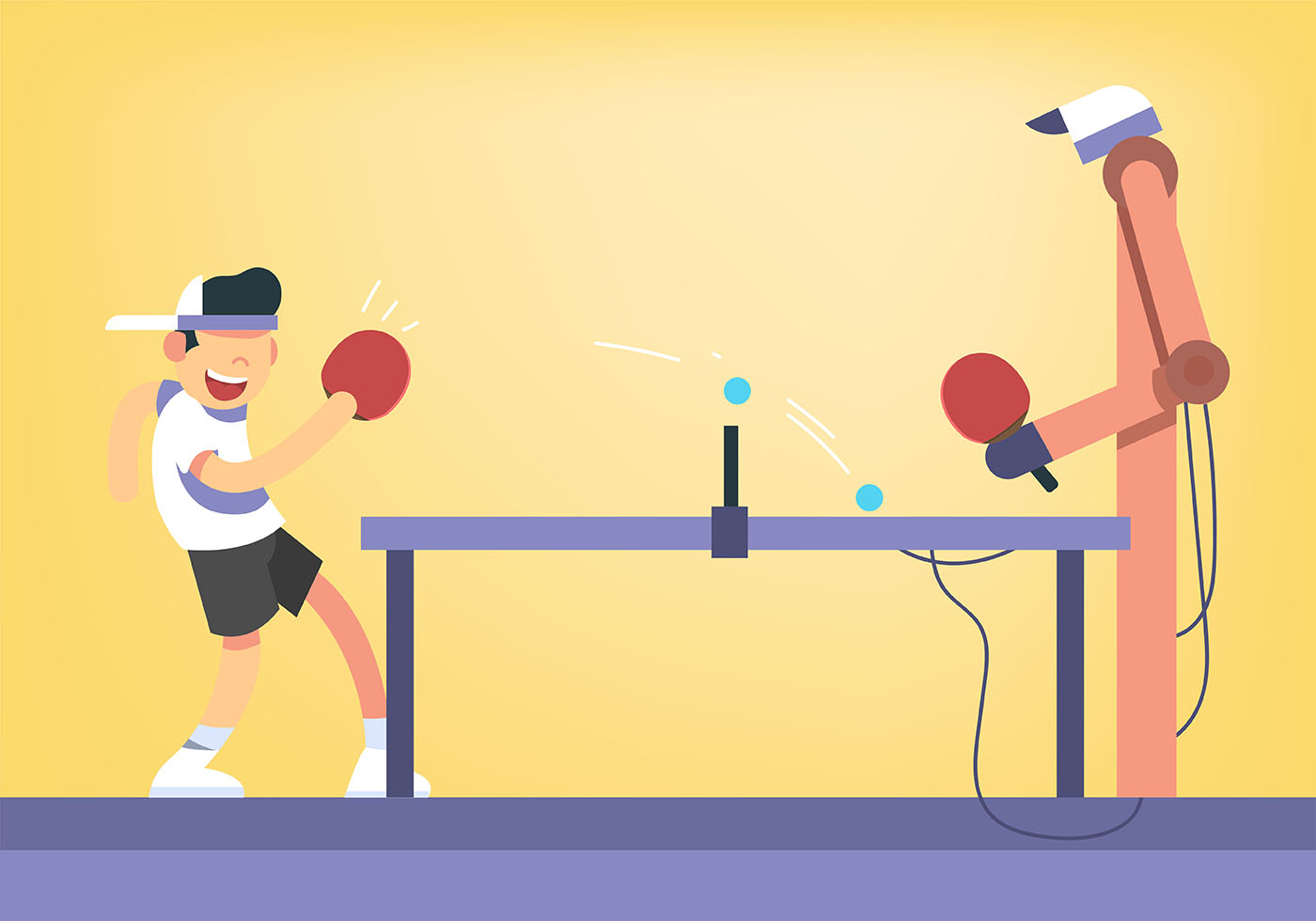
Probabilistic Graphical Models for Spatio-temporal Data
Spatio-temporal data are ubiquitous in science and engineering applications. We are pursuing a variety of techniques for modeling such datasets, mainly using probabilistic graphical models and other graph-based analyses. We primarily use these tools to enable the scientific analysis of, and predictive modeling from brain networks. Of particular interest are novel methods that address issues of confounding and multimodality.
Generative Models for Biological Images
Data in scientific and commercial disciplines are increasingly characterized by high dimensions and relatively few samples. For such cases, apriori knowledge gleaned from experts, and experimental evidence is invaluable for recovering meaningful models. Generative models are ideal for such knowledge-driven low data settings. We are developing a variety of generative models for biological imaging data and exploring novel applications of these models. We are also developing novel variational inference techniques that lead to scalable and accurate inferences, particularly for high-dimensional structured problems.
Learning with Aggregated Data
Existing work in spatiotemporal data analysis often assumes that data are available as individual measurements. However, for many applications in econometrics, financial forecasting, and healthcare, data is often only available as aggregates. Data aggregation presents severe mathematical challenges to learning and inference, and a naive application of standard techniques is susceptible to the ecological fallacy. We have shown that in some cases, the aggregation has only a mild effect on model estimates. For other cases, we are developing a variety of tools that enable provably accurate predictive modeling with aggregated data while avoiding unnecessary and error-prone data reconstruction.